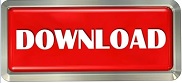
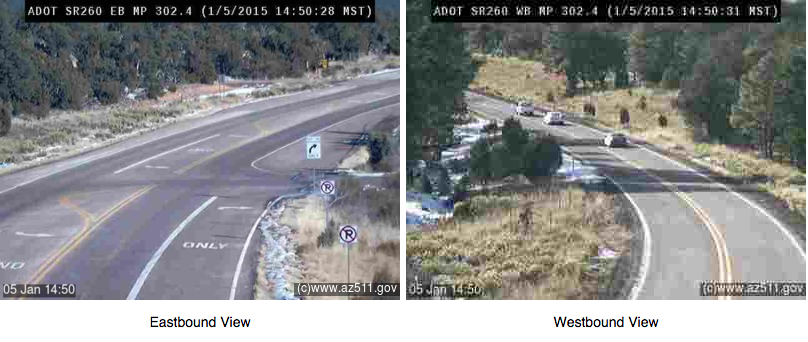
In many ways, the core technical challenge came down to building this dataset of visits. Once we had a dataset which associated clusters of GPS points to our safegraph_place_id key (we call each association a visit), we could simply “roll-up” the data by our key to build Patterns. To build this mapping between places and visit statistics, we first needed to build an internal dataset which associated our anonymous, internal GPS feed to the physical places in Places using our dataset of geofences. Use the coupon code data4databricksers for $100 in free points-of-interest, building footprint, and foot-traffic insights data. Some examples of columns we compute include the number of visitors, the hours throughout the day in which the place is most popular, and a list of other brands that people visited before or after visiting the place in question.Ī few columns of our Patterns dataset, from. Each row would be a unique physical place, and we planned to compute a set of columns for each place which collectively described how people interacted with it. Ultimately, we wanted Patterns to be keyed by safegraph_place_id, which is our canonical identifier for each place in our dataset. Safegraph datasets Patterns - how do humans interact with physical places? This post is about how we built Patterns, a dataset about the physical places around us and how humans interact with them. Below are SafeGraph’s 3 main datasets, each of which tells a different story of the physical around. This dataset is complemented by Geometry, a supplementary dataset that associates each place with a geofence to indicate the building’s physical footprint. One such dataset is our Core Places product, which is a listing of 5MM+ businesses around the country, complete with rich information like category and open hours. To serve this mission, we create datasets that represent the world around us.
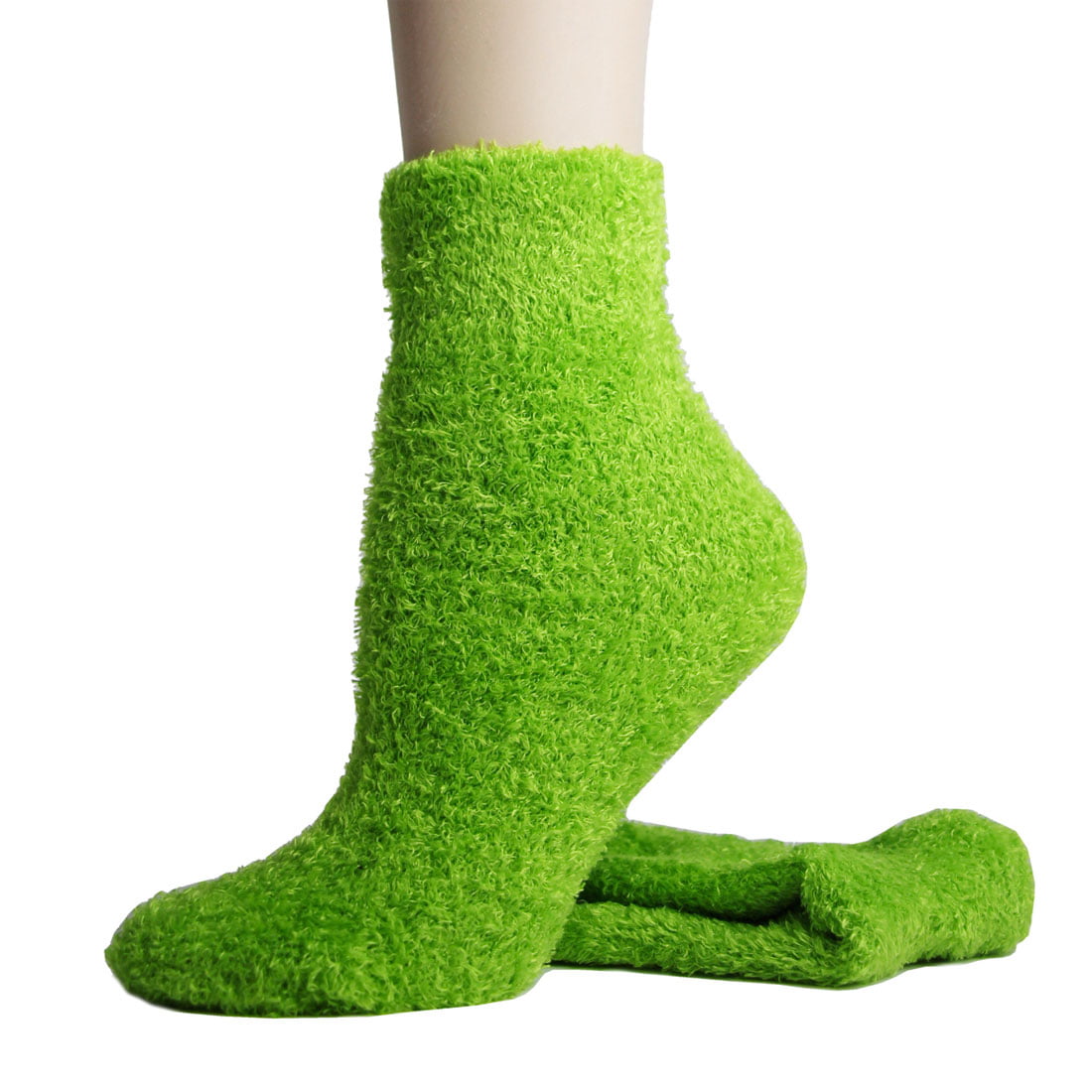
Our goal is to be the one-stop-shop for anyone seeking to understand the physical places around them - restaurants, airports, colleges, salons.the list goes on. And throughout this post, we’ll tell you exactly how we solved it.įirst, some context on SafeGraph. Building a dataset to answer these questions comprehensively is a massively difficult computation problem because it requires heavy machine learning at a significant scale. But they also hint at an interesting technical problem: machine learning at scale. These questions are no-doubt interesting for urban planners, advertisers, hedge funds, and brick-and-mortar businesses. Where should I build my next coffee shop? How far away are my 3 closest coffee competitors? How far are people traveling to get to my stores? Which other brands do people visit before and after they visit mine? Where should I build my next coffee shop?īusinesses want to understand both the physical world around them and how people interact with the physical world.
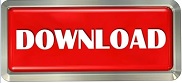